[ad_1]
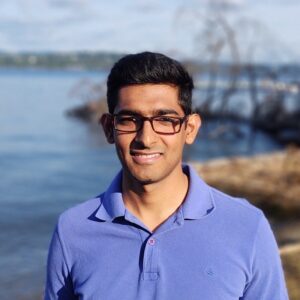
A new startup wants to make financial analysts more efficient with its chatbot tool that uses artificial intelligence to search publicly available business filings and answer research questions in natural language.
Finpilot is led by CEO Lakshay Chauhan, a longtime machine learning engineer at Seattle hedge fund Euclidean. He’s joined by John Alberg, the fund’s co-founder, who will play a close advisory role. Both Chauhan and Alberg are founding board members.
The startup recently landed $500,000 in a convertible security from Madrona Venture Labs and Ascend, both of which are investing heavily in AI companies.
“We were drawn to Lakshay and John’s deep understanding of the problems financial analysts face and the clarity of their product solution, which enables world-class research and reporting in a fraction of the time as existing tools,” Madrona Venture Labs Managing Director Mike Fridgen told GeekWire.
Alberg is the son of the late Tom Alberg, the Madrona Venture Group co-founder and Seattle tech community leader who died last year. Finpilot will operate independently of Euclidean, which uses machine learning to pick stocks and recently launched an exchange-traded fund (ETF).
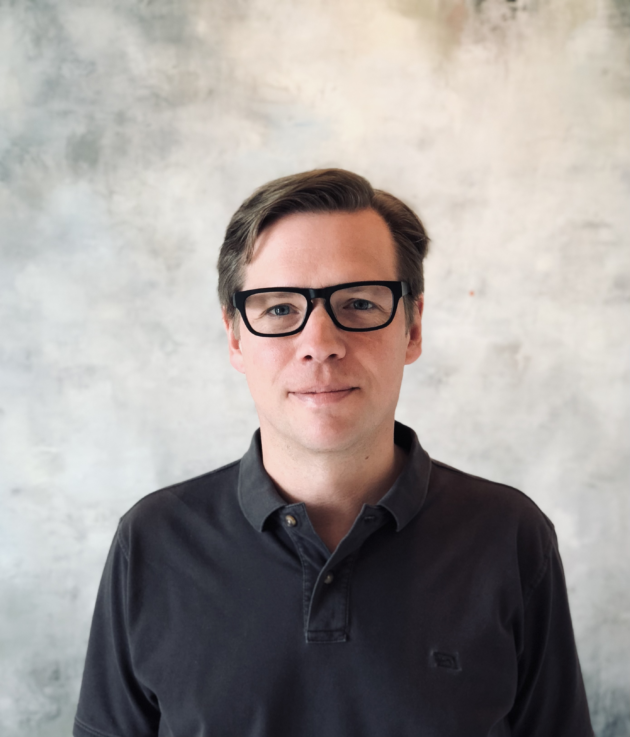
The startup is developing a ChatGPT-like interface. The tool uses a large language model (LLM) to scour publicly available financial data from sources such as Securities & Exchange Commission filings, earnings transcripts, and investor presentations.
This addresses a common pain point in the information-gathering process, where the traditional method involves relying on the Ctrl+F keyboard function to search filings, Alberg said. Using Finpilot, research and reporting projects that would otherwise take days or weeks could be accomplished within a few hours, he said.
Finpilot lets analysts write queries in natural language. For instance, a user could ask the chatbot about the increase in comparable sales for Walmart over the past 12 months. The tool would then produce an answer with links to the original source documents. Analysts can continue conversations with follow-up questions, gathering increasingly in-depth insights.
Alberg said Finpilot is unlike ChatGPT because users can interact with data, visualize it, and verify sources. The entrepreneur and hedge-fund manager has been a longtime proponent of using machine learning as an investment tool.
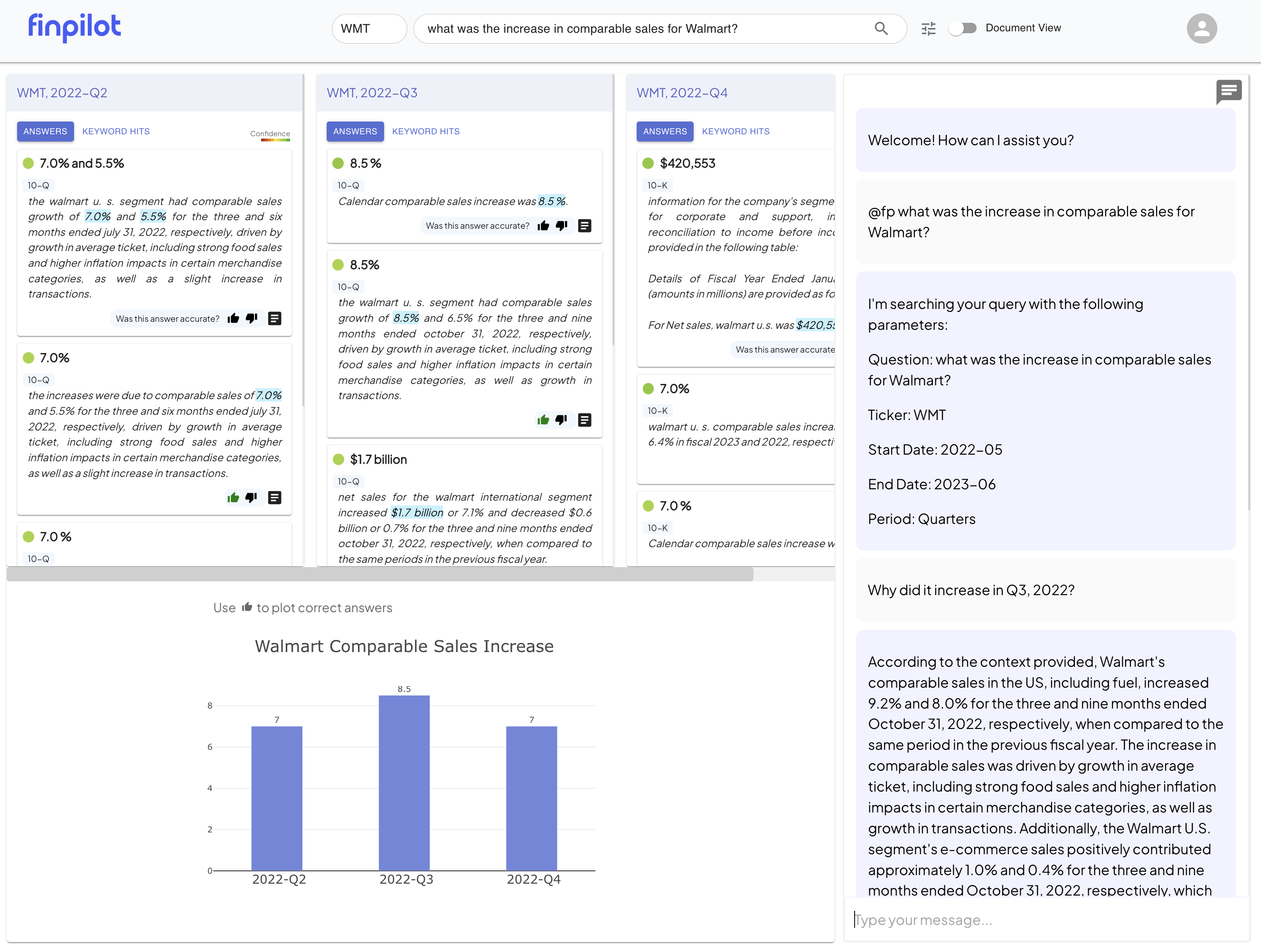
Finpilot’s technology is built on an in-house LLM specifically tailored for finance. It also uses some LLM-based APIs and a web-app stack for app deployment. The platform is in development and the plan is to sell it through a software-as-a-service (SaaS) license. The company is actively testing it with multiple customers, Alberg said.
Companies such as Factset, Bloomberg, and Alpha Sense offer financial data tools. The SEC’s EDGAR advanced search tool lets users search electronic filings dating back to 2001 using keywords and names. However, Finpilot helps analyze companies, write reports, and visualize data through natural language, Alberg said.
Madrona Venture Labs and Ascend invested in Finpilot through a SAFE note, or Simple Agreement for Future Equity.
The startup’s launch coincides with a rush of Wall Street firms investing heavily in artificial intelligence tools and expertise. Banks are betting that recent advancements in the tech can make data processing and risk modeling easier, gaining an edge as analysis becomes increasingly complex.
However, experts caution the widespread implementation of AI in finance may pose several potential drawbacks. A concern is the potential for AI chatbots to provide inaccurate information.
Traders, who have a fiduciary duty to base investment decisions on reliable data, might face legal uncertainties when using AI. Tracing the training data of chatbots can be challenging, hindering the ability to identify the source behind a false data point used to make an investment decision.
This will be a challenge for Finpilot, but the company aims to provide users the “highest accuracy, speed and reliability,” Alberg said.
“Large language models are notorious for making up information and are too slow for many tasks,” he said. “We developed many techniques to address these issues.”
[ad_2]
Source link